AI Room Makeover: Reskinning Reality With ControlNet, Stable Diffusion & EbSynth
Artists have wished for deeper levels on control when creating generative imagery, and ControlNet brings that control in spades. Let's make a video-to-video AI workflow with it to reskin a room.
Hey Creative Technologists! Today we’ll be covering an AI video experiment I created to learn and prepare a deep dive on ControlNet.
Motivation: Why we need more than text2img and img2img
Let’s face it… text is a pretty fickle way to describe the intricate composition of a scene, the lighting conditions, the pose of the characters, the objects within in the scene etc. Typically artists need to go through hundreds of iterations, using a myriad of unspecific negative prompts (bad anatomy anyone?) to get a satisfactory final result.
Mood boarding is one thing, but what if you have a very specific creative vision in mind? Enter ControlNet — game-changing method (by two grad students!) that allows artists to take large pre-trained diffusion models (like Stable Diffusion 1.5) and extend them to support additional input conditions.
These input conditions (e.g. depth maps, full body pose, edge maps, normal maps) give artists new ways to exert control over the otherwise chaotic diffusion process. And once you compose them together, magic happens:
As always, the best way to learn about a new capability is to use the darn thing to make something — so I gave myself a challenge to create a video animation reskinning a room.
Okay, now let's quickly break down this hacky but fun workflow!

1/ INPUT VIDEO: DEFINING THE SCENE LAYOUT & CAMERA ANIMATION
For my input, I made an animation from a photogrammetry 3D scan I did a few years ago of my parents' living room in India. After setting up interest points in SketchFab, I simply screen recorded a 3D viewer while I animated the camera from point to point.

2/ DEPTH MAP GENERATION: DESCRIBING SCENE STRUCTURE FROM THE CAMERA'S POV
I used MiDaS to generate depth formatted correctly to work with ControlNet. It's a SOTA model that can infer depth using a single 2D photo as an input on the clip. For an indoor scene like this it'll do great. Quick and simple.

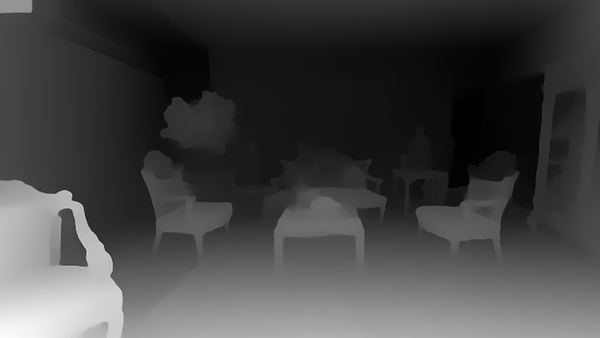
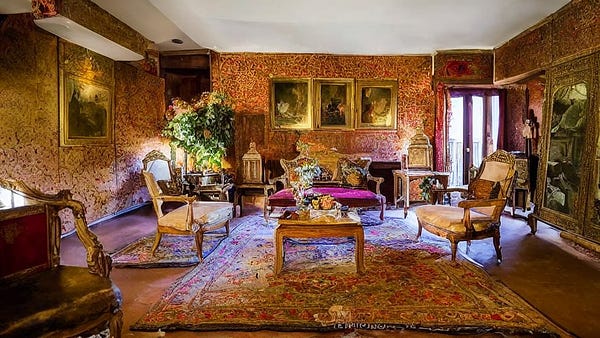
3/ CONTROLNET + SD 1.5: CONTROLLING THE CHAOS WITH DEPTH CONDITIONING
Depth conditioning is sweet, and produced stunning results reskinning this room. The higher res depth guidance makes it easy to nail in the contemporary look I want because a lot of that is encapsulated in the furniture. Not only is CN high res vs. SD 2.0 (while being cheaper to train!) it brings depth conditioning to SD 1.5, which many artists still prefer.
I’ve covered Depth2Image extensively, so be sure to check out these posts if you’re interested in why it’s important and the creative use cases it enables:
4/ MAKING IT SMOOOOTH WITH EBSYNTH + AFTER EFFECTS
I generated 16 keyframes where I toggle the styles, and ran it through EbSynth to create a temporally coherent end result across the 300 frame clip. EbSynth uses "non-parametric example-based synthesis" to propagate those keyframes (classical CG ftw!). But you will definitely need to bring the final result into your video editor to blend and composite to to be less jarringly between keyframes.
5/ TAKEAWAY: MIX METHODS FOR BEST RESULTS WITH COMPLEX SCENES
Unsurprisingly, different ControlNet methods have their pros and cons depending on your subject matter and they will need to be tuned.
For instance the example below compares processing the same video at 30fps with two different ControlNet methods. "Canny" does well in keeping the wall paintings consistent (thanks to the contrast-y edges), but struggles with the table. Meanwhile, "Depth" crushes it with the furniture but turns the walls into a flickering mess (since it's flat w/ no depth cues).

My next experiment will be to combine the strengths of various ControlNet methods into one composite, which will produce even better results for a video2video pipeline.
Closing Notes:
I plan to share all my findings in a ControlNet deep dive post later this week! Subscribe to get the deep dive right to your email:
Twitter fam — Clearly ya’ll want equal parts tech + creativity :)
Want more visual umami? Check out a highlight of my top tweets here here, something which I plan to keep updating periodically.
Got feedback on topics you’d like me to cover, or just want to get in touch? Connect on Twitter or drop me an email here.
Cheers,
Rudimentary footage is all that you require-- and the new software’s and the creator can weave magic.
If you already got a 3D scan in step 1, why did you use MIDAS to estimate depth in step 2? 3D scan essentially tells you the depth right?